Hazard: Reinforces Existing Biases#
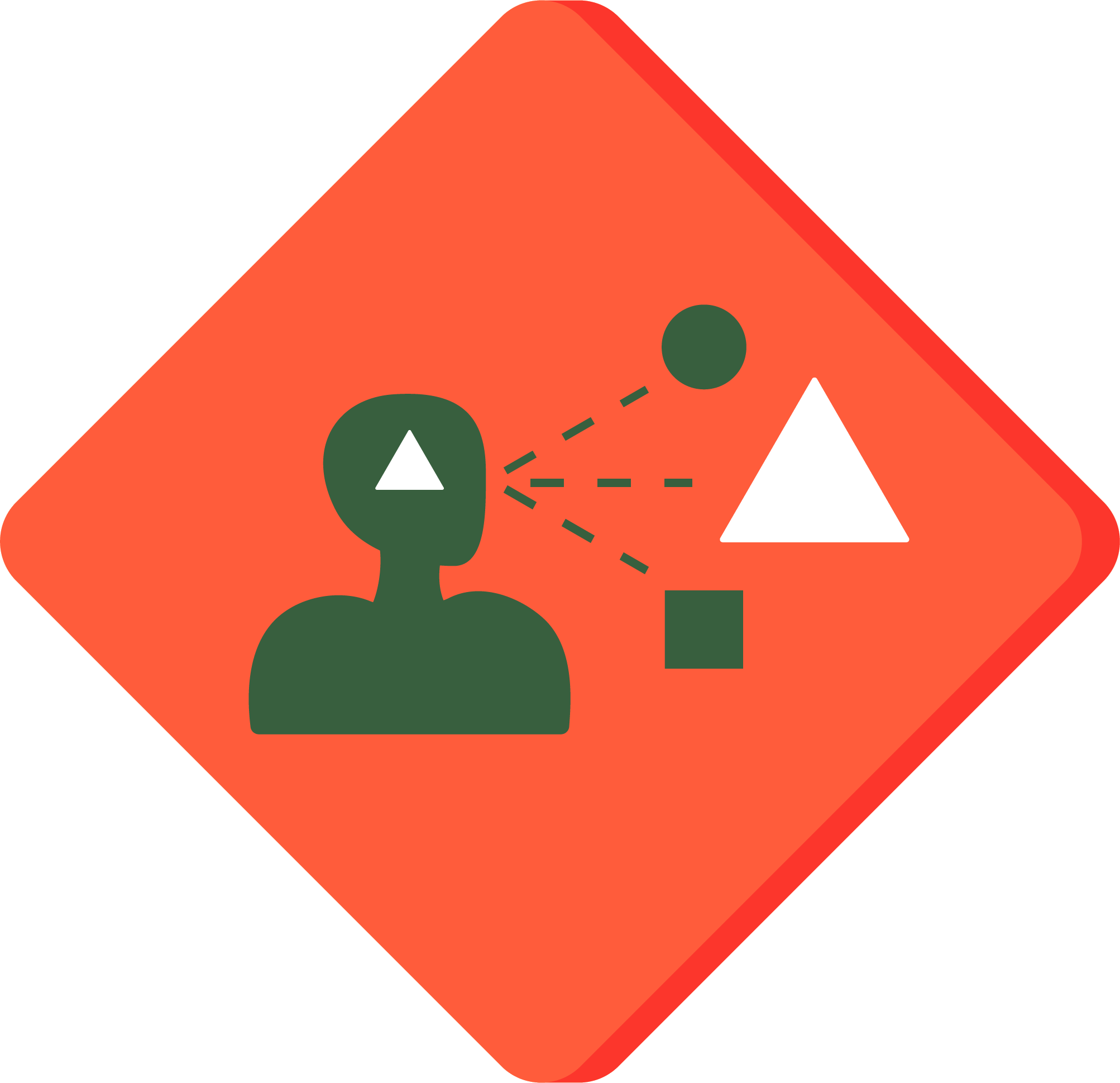
Description#
Reinforces unfair treatment of individuals and groups. This may be due to for example input data, algorithm or software design choices, or society at large.
Note: this is a Hazard in it’s own right, even if it isn’t then used to harm people directly, due to e.g. reinforcing stereotypes.
Examples#
Example 1: Natural Language Processing tools can reinforce sexist tropes about women.
Example 2: Automated soap dispensers that do not work for Black people
Example 3: UK Passport facial recognition checks do not work for people with dark skin
Safety Precautions#
Test the effect of the algorithm for different marginalised groups, considering different definitions of bias and fairness.
Think about the input data, what intrinsic bias it contains, and how this can be reduced (for example by having a more representative data set).
Think about the bias of the algorithm, what intrinsic bias it contains, and how this can be reduced.
Do not deploy tools that are know to reinforce biases against particular groups (for instance, systemic racism).